“AI is amazing at guessing quickly, but it fundamentally can’t reason.”
That is a quote from someone I know very well, circa 2022. I wonder what the thought process was; the reasoning that went into that statement. Luckily I don’t have to guess, because that person was me. I made that statement in response to the first rounds of LLMs (GPT3, 3.5, PALM2 etc). It remained a firm conviction of mine through the release of GPT-4o and Anthropic’s latest Claude models.
Both proved incredible at resolving problems, but only if they were presented with all the facts, and subsequently, guided through the steps. We were “patching” the reasoning process with hacks, such as prompt engineering, RAG systems, multi-agent conversations, chain-of-thought prompting, and so on.
At Tricentis, our team is working day to day implementing LLMs to solve complex multi-step problems, so we were at the coalface, seeing just how unreliable these solutions became in practice. Frustrating days passed, as we tried to figure out “Why wont the AI do the obvious next step!?” Or, as we added more and more complex prompts, to shoehorn predictability at the cost of generalized usefulness. At every turn, the conviction that AI couldn’t reason became more concrete in my mind.
Until now. So what changed?
On Jan. 20, the Chinese AI company DeepSeek released a language model called R1 that, according to the company, outperforms industry leading models like OpenAI o1 on several benchmarks. These two models fit into a new class: Models designed, and trained, to reason. Let’s dive into why that matters, and why DeepSeek R1 specifically has sent shockwaves through the AI industry.
Traditional LLMs can’t reason
The way traditional language models (strange as it may sound to say that about a technology all of 8 minutes old) are trained is generally in two ways:
1. Fill in the blank
A common training method involves masking words in a sentence and having the model predict what those words should be. This is a form of unsupervised training, since no human intervention is needed, and is the baseline of all LLMs. By showing them massive amounts of text, where certain words are obscured, they learn to predict what words fill that gap based on the surrounding text. It’s a massive oversimplification, hiding the complexity of attention heads and tokens, but essentially this allows them to learn the manifold meaning of language, in context, not just the dictionary definitions.
2. Reinforcement learning with human feedback (RLHF)
Human reinforcement, or RLHF, is a supervised technique, often used for fine-tuning after the initial training. These teach the AI to get better at giving the “type of responses humans expect.” Yes, that’s a vague phrase, but we are vague beings. Here, we tune the LLM to answer questions, respond to conversation, and offer feedback that improves subsequent responses. We basically teach it to be a chatbot, and as a by-product it learns to approximate solutions to some problems, and to deliver them in language that its trainers have deemed socially acceptable.
This is not how we solve complex problems
Think about how you would solve a complex problem. This could be anything from designing an application feature, to writing this blog post. You plan, you build, you iterate, you correct, you conclude. It is a multi-step process, involving continual reflection: Am I doing this right? Is this the right track? Do I need to adjust?
This is where traditional LLMs fail. They are trained to give the answer in one quick shot.
Thinking fast and slow
In his book “Thinking, Fast and Slow,” Daniel Kahneman proposes two models for how we think:
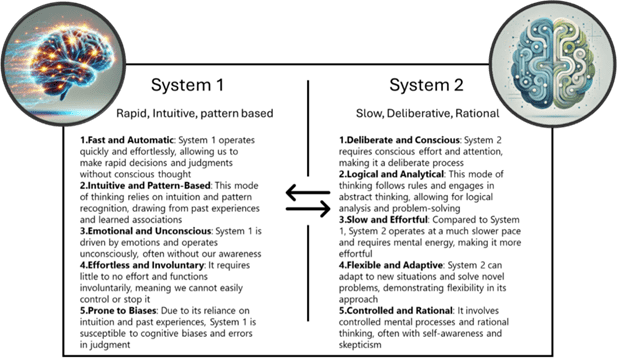
These map really well to the patterns of language models. All the models released prior to OpenAI’s o1 model were essentially System 1 models. They responded fast, matched patterns, and did their best based on what you gave them.
Again, what changed?
OpenAI o1 and the introduction of System 2 (reasoning) models
OpenAI is not Open AI
When OpenAI launched o1 in September of 2024, they titled the post “Learning to reason with LLMs.” The results in benchmarks were pretty impressive, especially in complex tasks:

I found it particularly fascinating that it showed no improvement in AP English, demonstrating once and for all to my high school English teacher that English is irrational.
But OpenAI only published the what. They gave very little information as to the how. This was something of a competitive differentiator. Claims of massive compute resources needed, cups of water vanishing for every request, and power requirements needing entire nations to redo their energy grids fed the Venture Capital machine to push more and more money into these heavily funded, transparent-as-mud AI enterprises, seeing the promise of human-level reasoning models finally coming to fruition.
Evidence of System 2 “thinking:” The shift to test-time compute
O1 was the first model to shift the way models responded to questions. Instead of immediately launching into the answer, like a nervous intern at a job interview, they began to think first by design. When answering a question, the model will immediately begin an internal monologue, planning out its actions. When OpenAI launched o1, they called this “the hidden chain of thought,” and like everything else OpenAI does, the techniques were hidden along with it. The result, however, was that the o1 series of models began to output step-by-step complex plans to solve difficult problems, achieving impressive results!
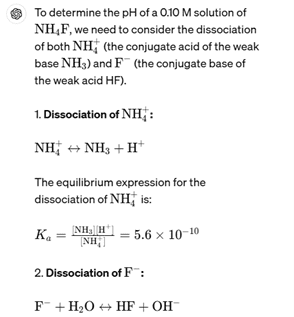
Partial o1 response to a chemistry question
This was the shift away from train-time compute, where the model learns the patterns and responds ‘instinctually,’ to test-time compute, where the model responds far slower, with more compute required (driven by that ‘internal monologue’) before giving a thoughtful, planned-out answer.
Why does DeepSeek change the game?
DeepSeek is Open AI (sort of)
When DeepSeek published their paper on how they trained DeepSeek R1, they published training techniques, experiments, ablation studies (comparisons with state of the art), failures, future experiments, quantization and optimization methods. When they published their model, it was ready to be fine-tuned, easily accessible on Hugging Face, and open for use. It was published under an open MIT License, meaning it can be used commercially and without restrictions.
What DeepSeek didn’t publish is the dataset they used to train R1. That remains closed. But speculation abounds that they used OpenAI models to train and fine tune their own. Aside from that minor detail, R1 is a very transparent AI release, which allows enterprises and researchers to experiment with and train powerful reasoning models on their own use cases – potentially at a significantly lower cost, and with significantly fewer resources.
DeepSeek is cheap and fast
Alongside its full-size model, DeepSeek also released distilled versions of R1, or quantized models, that have been optimized to run on consumer hardware, opening up the opportunity for edge LLM and calling a major AI industry assumption into question – that the best way to make AI models smarter is by giving them more computing power. Benchmarks are still pouring in, but this Reddit post is replete with examples of models running on Mac M3, or consumer-grade Nvidia chips.
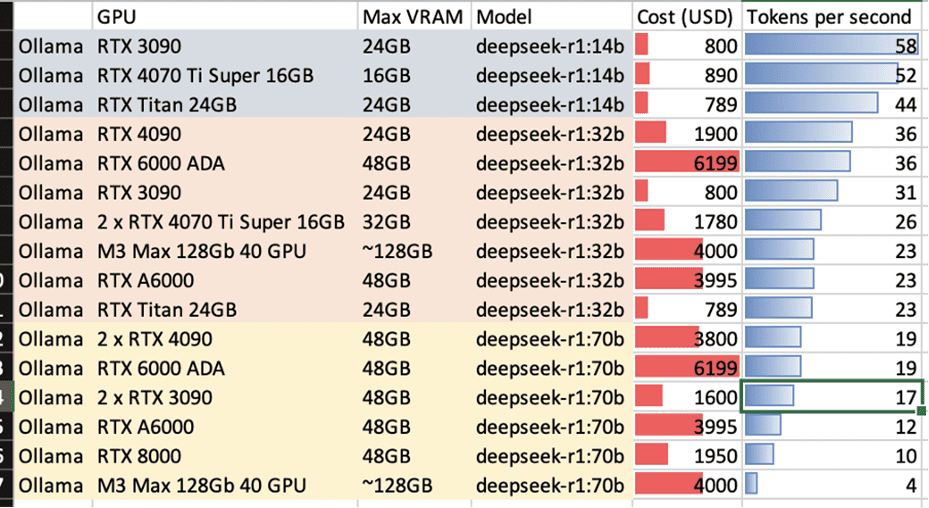
This may be the reason behind the massive sell-off of Nvidia stock; however, it should be noted that DeepSeek has access to a massive number of Nvidia H100 chips, at a lowball retail estimate of $1.5 billion, so training models is still the domain of the well-funded. It has been widely reported that DeepSeek spent $6 million on the hardware used for R1’s final training run. I would be shocked if the total cost was less than $50 million, so take the hype here with a small trailer-load of salt.
DeepSeek demystified reasoning
Most importantly, DeepSeek contributed the “how” of reasoning models back to the general public, allowing researchers, startups, and giant tech companies to train their own reasoning models, on specific use cases. They also provided invaluable lessons learned, here are a few of my favorites, taken from the DeepSeek paper:
- By incentivizing the model to think first, but not teaching it what to think, the model naturally learned how to expand the amount of time spent thinking prior to answering, which led to better solutions in more complex problems:
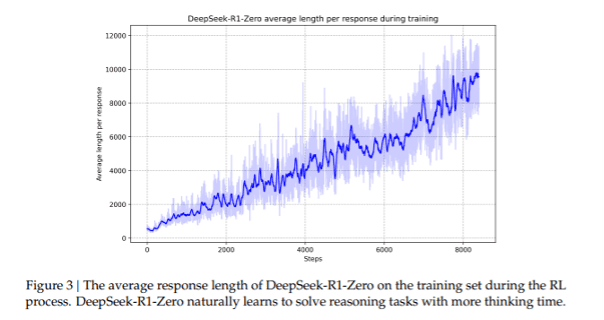
- Without prompting, the model learned to “rethink,” revaluating its path and identifying and correcting mistakes in reasoning. Since what we get from the model is essentially a stream of consciousness, it was almost charming how “humanesque” it was when identifying its mistakes:
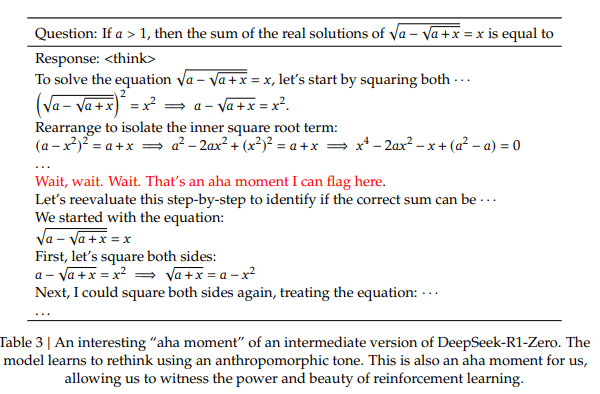
- Guidance (cold start data) is still required to “humanize” the outputs. Without it, the model was completely happy generating its chain of thought while swapping between languages and formats, but it was absolutely unreadable. A little example data went a long way here.
Price at the cost of privacy
Much of the panic in the AI market has been driven by the fact that DeepSeek is offering its full R1 API at pennies on the dollar compared to OpenAI:
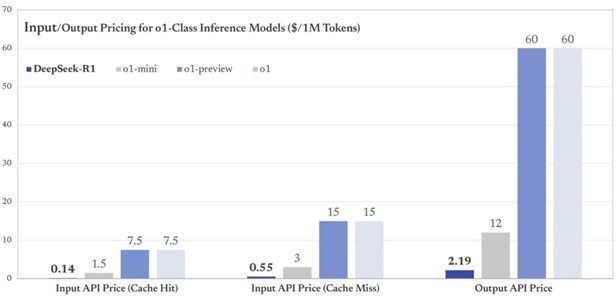
API pricing comparison between DeepSeek-R1 and OpenAI models by DeepSeek API Docs
Yes, those invisible bars are DeepSeek prices. But there is a heavy hidden cost here.
One thing DeepSeek is not open about is how they use, store, and manage the data that you send to them. Reports of it collecting keystrokes, prompt, audio, and video data and open up legitimate concerns about how that data is used, for what purpose, and by whom. I am not about to wade into the murky land of geopolitics, but suffice it to say that you should talk to your organization’s legal counsel and data privacy team before you even send your first prompt. For reference, this is the reaction that mine gave:
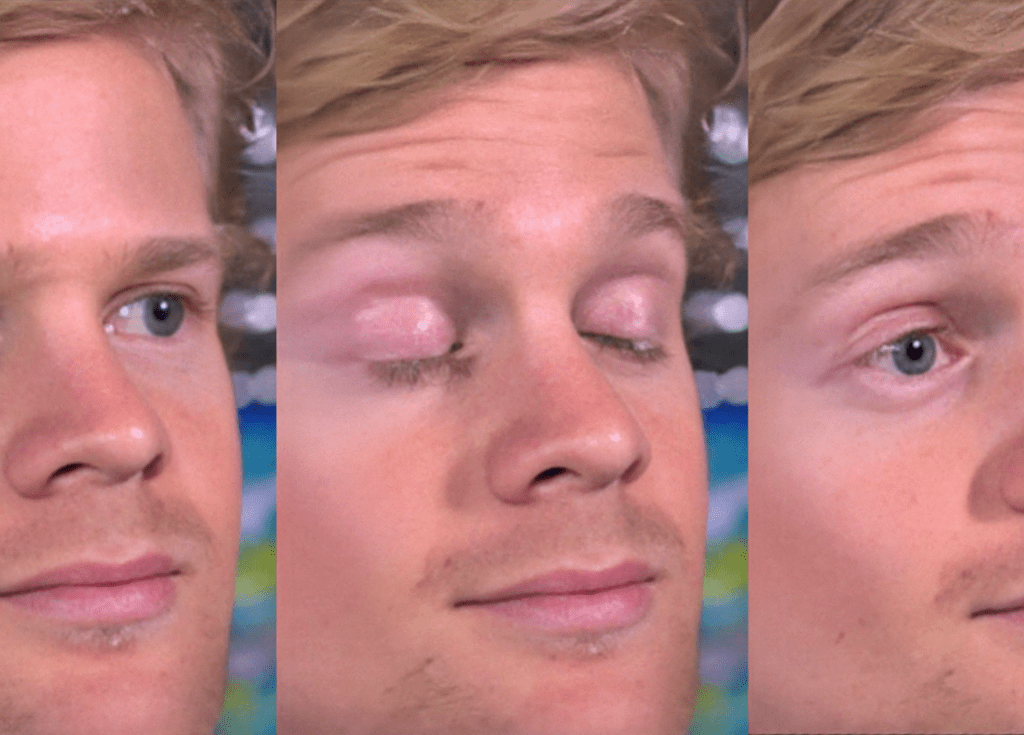
But what about o3?
This is true; the DeepSeek models are not as good as the o3 models, but I am convinced that they will be. I am convinced of it because the template that they provided for how these models are trained gives a low-cost path towards incrementally improving, specializing and deploying these models for tasks such as coding, math, and science. We will see the next version of DeepSeek within months, and the open research alternatives and improvements are already popping up.
The moat built around OpenAI’s reasoning models has been bridged, and the game is afoot.
What does this mean for QA and DevOps?
These are planning tasks
Current models are doing alright at dev and QA tasks. They are proving good at generating code, or proposing tests, but they have a shortcoming. They perform poorly when the task requires thinking one or two layers deeper. For coding, this might be considering design principles or planning out an API library before implementing. For QA, this takes the form of applying testing strategies, considering techniques such as boundaries, security loopholes, confounding variables, and combinations of inputs that could provoke defective behavior.
O1 models are good, but most of the data is confidential
At Tricentis, we have seen a strong reticence in our customers towards fully adopting cloud-based, opaque models. They may trust us, but we are asking them to also relay that trust on to a third party (OpenAI). This is a bridge too far for many data-savvy enterprises or groups that have heavy regulatory or privacy burdens.
DeepSeek opens the door to custom, private, edge AI
DeepSeek provides the ideal blend of performance (being a competent reasoning model), adaptability (through fine tuning, with models like DeepSeek Coder already popping up), and Deployability. I see this being a game changer for the highly sensitive world of development and QA.
Closing thoughts
If you made it this far by reading the whole article, thank you. If you skipped to the end to get the conclusions, here they are:
- DeepSeek is a game changer for the open source community, providing bleeding edge performance without the opacity of API deployed models
- Reasoning models are the next phase of AI development, and thanks to this open research will accelerate faster than I had thought possible
- AI that can think is here, and the types of tasks it can do are fairly unbounded
- DeepSeek™ still has some serious privacy problems, so handle with care If you are interested in how we use LLMs to solve problems in the testing and DevOps space, you can explore our Copilot solutions here or feel free to connect with me on LinkedIn.
Author: David Colwell
VP, AI & Machine Learning
Date: Jan. 30, 2025